Leveraging Graph Databases: Top 5 Applications
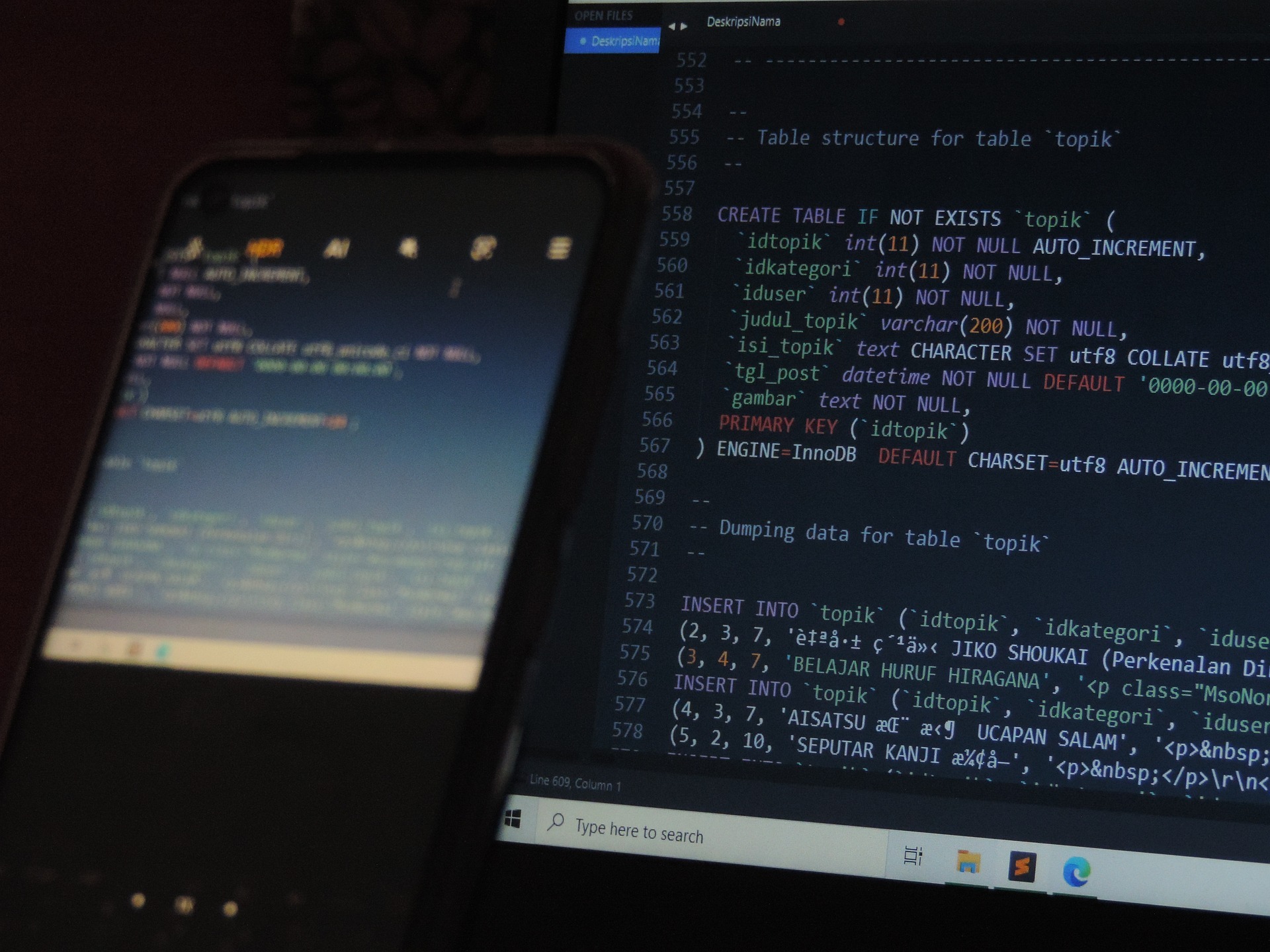
Graph databases have become one of the most significant technological advancements to come out of the NoSQL database revolution. These databases were created to extract insights from large amounts of interconnected data, by storing the relationships between data objects within the objects themselves. This allows for incredibly fast analysis, which would be difficult to achieve with other methods.
Graph databases are intended to complement relational databases, rather than replace them. They provide the capability of performing complex queries quickly across data from multiple systems, without the need for table joins or data transformations. This can be achieved by integrating data from multiple sources, often through a data lake.
The advantages of graph databases go beyond query speed. Complex relational models can be modeled easily and schemas can change dynamically. Additionally, SQL-fluent users need not feel left out as graph database query languages such as GSQL, are SQL-adjacent languages augmented with graph capabilities.
Furthermore, the focus on relationships and the ability to handle large amounts of data efficiently make graph databases an ideal fit for AI and ML applications. This can be further enhanced when the graph database software includes AI/ML-specific tools and interoperability features.
With so many capabilities, it’s no surprise that various industries have begun to adopt graph databases. Here’s a look at how five industries are utilizing the extremely fast relational query performance across distributed data stores.
1- Unlocking the full potential of customer interactions
Interactions between businesses and their clients or potential buyers tend to be intricate, with numerous points of contact. Ideally, these interactions should result in sales strategies that are continuously tailored to meet customer needs. However, these 360-degree scenarios often result in many-to-many relationships that, when using a traditional relational database, would require extensive modeling and complex table joins to yield valuable insights.
This is where a graph database comes in. A prime example is UnitedHealth Group (UHG), the largest healthcare company in the world by revenue, which has adopted a graph database to improve the quality of care for over 26 million members while reducing costs. UHG uses a massive graph database to track more than 120 billion relationships among members, providers, claims, visits, prescriptions, procedures and more.
UHG has created various user interface applications that use its graph database to provide a consolidated view of member interactions with physicians, pharmacies, clinical labs, health advisors, and UHG itself. Over 23,000 users access the database daily, allowing providers to make better care and wellness recommendations based on the latest member activity in real-time. UHG predicts that the cost savings may eventually reach billions.
2- Revolutionizing financial services with AI
The rapid growth of data has been the driving force behind the development of AI/ML, which requires vast amounts of data to surface meaningful patterns and improve the accuracy of decision-making. Among the industries that generate the most data is financial services, but data from various sources often ends up in silos within relational databases.
Graph databases can bridge these silos, allowing AI/ML to deliver superior predictive analytics, risk management, fraud detection, anti-money laundering, insider-trading monitoring, automated recommendations for customers, and more. Furthermore, a graph database coupled with AI/ML can ensure data is accurate in the first place, resolving anomalous differences in customer records and financial product attributes that could lead to inaccurate results.
Intuit, for example, is using graph database software in combination with AI/ML to transform from a product-based company to an AI-driven expert platform company. A key aspect of this transformation is the creation of knowledge graphs, which enrich data and surface insights from clusters of related elements. Intuit combines knowledge graphs with deep learning, the most advanced form of ML, to power its chatbots and in-app recommendations. An added benefit of Intuit’s knowledge graphs is that they add “explainability” to deep learning outcomes.
3- Streamlining logistics
The COVID-19 pandemic has highlighted the vulnerability of global supply chains, and manufacturers are now more aware than ever of the complexity of maintaining and optimizing them. One industry that has felt this impact is the automotive industry. Auto manufacturers must accurately predict customer demand to determine the types and quantity of parts needed, taking into account various models and options buyers may choose. These predictions must also align with the availability of parts from numerous suppliers, as well as factors such as manufacturing efficiency and supplier risk.
To tackle these challenges, Jaguar Land Rover (JLR) turned to a graph database solution. This technology allowed JLR to access multiple data silos for supply chain analysis and explore the relationships between data elements. The goal was to increase profit per unit sold, decrease aged inventory and minimize the impact of supplier disruptions. As a result, some key supply-chain planning queries at JLR now take only 45 minutes, compared to weeks previously, and management can now answer questions they were previously unable to.
4- Enhancing ecommerce
Ecommerce companies are under increasing pressure to provide a superior customer experience, which relies on accurate customer information and purchase history. This information is crucial for dynamic pricing, product recommendations, and personalized special offers, all of which draw on data collected throughout the customer journey.
Graph databases can assist in various ways, such as identifying relationships between customers and payment methods, customers and brands, products and return rates, promotions and sell-through rates, among others. For example, a query to determine which promotions were most effective for a certain product when pitched to a certain group of customers can be run quickly and efficiently with a graph database, compared to a traditional relational database. Additionally, a graph database can aggregate and reconcile all customer data regardless of the payment method or point of sale, making identifying customer purchases more reliable. One ecommerce company found 12 million new account connections across its five websites in a three-month test of a graph database, leading to an estimated efficiency saving of nearly $3 million and a predicted 17.6% increase in sales.
5- Enhancing the precision of fraud detection
As we all know, fraud detection has undergone significant advancements in recent years, particularly in the banking, credit card, and telecommunications industries. Initially, rule-based systems were prone to overlooking suspicious transactions or falsely identifying legitimate transactions as fraudulent. However, with the integration of graph databases in AI/ML operations, the precision of fraud detection has significantly improved.
By combining AI/ML with graph databases, the detection of fraud is made more accurate, resulting in fewer false positives and the ability to identify anomalies that might have gone unnoticed. Machine learning requires a wide range of data types to model a customer’s typical behavior, such as location, device, payment method, and authentication method. Additionally, the definition of normal behavior patterns must be adaptable to changes in real-time. Graph databases provide this flexibility and enable AI/ML to analyze customer interactions to identify significant variations.
Leading financial institutions such as JP Morgan Chase and Intuit have implemented graph databases to boost their AI/ML fraud detection efforts. JP Morgan Chase uses a graph database to protect over 60 million households in the US. Intuit has reported that their graph-based machine learning has allowed them to detect 50% more potential fraud events and has reduced false positives by a similar percentage.
These are just a few examples of the various applications of graph databases. They are also being used to optimize business processes, improve healthcare outcomes, refine digital marketing strategies, identify cybersecurity threats, and even manage energy grids. New uses for graph databases are constantly being discovered.
The purpose of a graph database is to provide new perspectives on the relationships between data elements and to deliver analytics that can reveal new business opportunities, identify inefficiencies, and provide a versatile foundation for AI/ML projects. When connected to multiple enterprise data stores, graph databases can offer unprecedented insights and capabilities.